Open source content and tools at the core of automating complex process
City Environmental Quality Review, or CEQR, is the process by which New York City agencies determine what effect, if any, a discretionary action they approve may have upon the environment. CEQR is a disclosure process and not an approval process in and of itself. Completion of an environmental review supports other decisions made by agencies such as approvals of rezoning or variance applications, funding, or issuance of discretionary permits. Ministerial actions, such as the issuance of a building permit, are not subject to environmental review.
Historically, CEQR, along with other government environmental review programs such as the New York State Environmental Quality Review Act (SEQRA) and the National Environmental Protection Act (NEPA) have been the subject of much debate – right or wrong – with regard to being overwhelming, complicated, and costly to those individuals and/or organizations involved in projects or “actions” which trigger the application process.
CEQR is precursor to ULURP (Uniform Land Use Review Procedure), which, in part, is the approval process that decides the fate of the action. ULURP cannot start until the environmental review process is complete.
Introducing AutoCEQR
In the New York CEQR space, leave it to a couple seasoned GIS folks to step in and combine professional experience with geospatial tools and programming skills to offer a cost effective and streamlined process to work through the CEQR application.
AutoCEQR cofounder Matt Sloane has worked in the planning field since 2007, working extensively with SEQRA and CEQR. Over that time Matt developed specialties in both GIS and Data Science. As Matt learned to program the tools that power ESRI ArcDesktop software, he realized that many of the processes required by CEQR, which are explicitly prescribed by the CEQR Technical Manual, could be automated based on existing data (e.g., MapPLUTO) and several project-specific inputs. He approached Danny Sheehan, a close friend and former classmate at SUNY Geneseo’s planning and geography courses, about the project. Both agreed it would be a great opportunity to put their combined skills to work and build a platform to augment the CEQR application process. Danny was able to bring geospatial development expertise and software production knowledge he learned at UBS, Carto, and Columbia University to start and evolve the project into a production application.
AutoCEQR leverages a mixture of City, State, and Federal data resources, though primarily relies on NYC Open Data. Other data sources include:
- NYC Bytes of the Big Apple (NYC’s MapPLUTO data is hosted there)
- NYS GIS Clearinghouse
- National Park Service Open Data
- U.S. Census Bureau Census API
- Web scraping tools for some datasets (i.e. hotels, other locational datasets, etc) have also been developed
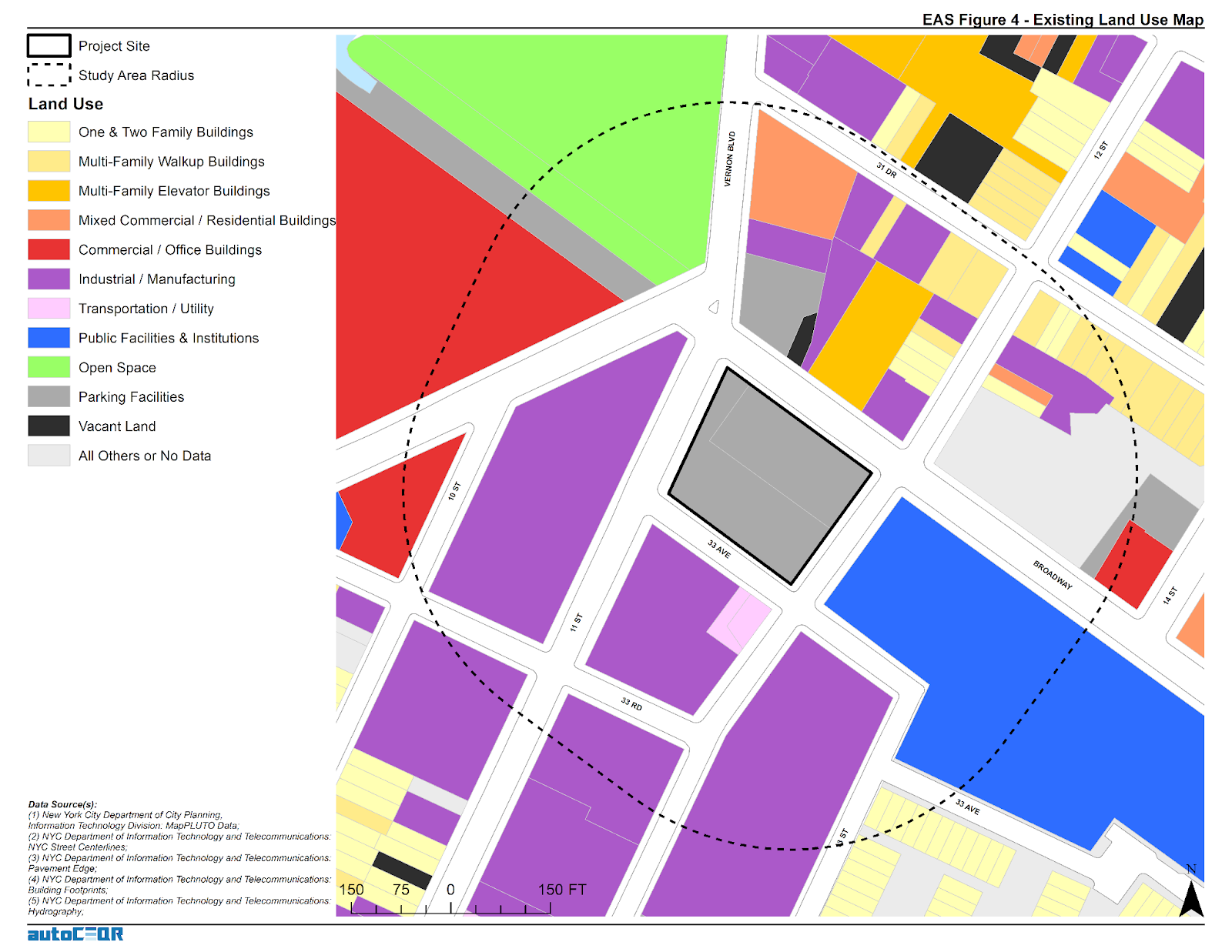
This 400’ radius buffer around a subject property which requires CEQR shows adjacent parcel land use classifications that are included in the NYC MapPluto file on a regular basis
A. Coding and Software Environments
Python is at the core of the AutoCEQR technology. For working with data, the AutoCEQR team uses Pandas, GeoPandas, Shapely, Fiona and ArcPy for generating Map Document files (.mxd’s), and creating custom Python classes for the workloads. Sheehan notes “With GeoPandas and Shapely it’s phenomenal how close to parity they now are for matching ArcPy functionality.” In the development environment, PyCharm Community Edition and GitHub are used for code development & versioning.
AutoCEQR prototyping started with ArcPy for all tasks but it was decided to abstract the high-level functions so the geoprocessing engine could be changed to GeoPandas, the geoprocessing library of choice. For interacting and communicating with Amazon Web Services (AWS) – the current AutoCEQR Cloud Computing Platform – developers leveraged Boto3 (AWS SDK for Python). EC2 and S3 is leveraged in the AWS environment for computing, data storage, and distribution which has enabled to keep the application computing bill fairly low per month. In the future, it is anticipated to modify the architecture by leveraging more serverless technology and more scalable architecture for added compute cost savings. AWS generously provided AutoCEQR with free computing credits for one year through AWS Activate – which was brought to their attention as part of their involvement and experience at the Columbia Startup Lab (CSL). QGIS is also used to verify results and quick GIS work.
Interacting with Census data and a whole host of services is made possible by leveraging the many great open-source libraries available on PyPl and GitHub. The storefront is the Squarespace AP which is used to process and deliver orders.
AutoCEQR still uses ArcPy mapping for generating maps, .mxd’s, and map packages but given the high cost of licensing and the technical slowdown it adds to both the production application and ongoing development speed, and it’s unclear if .mxd’s will exist in future iterations. (Both Sheehan and Sloane would like to have more feedback from users if the .mxd deliverable is necessary or if the application should generate static maps with Matplotlib and GeoPandas or if interactive web maps would be more helpful.)
The data engineering ETL process mostly consists of pulling down data with requests, unzipping files, some transformations and projecting data, and API libraries and a scheduler. We download the latest data every night – whether the source is updated daily or not. Data ETL would be a big focus to redesign to improve the platform and save on cloud storage and computing costs.
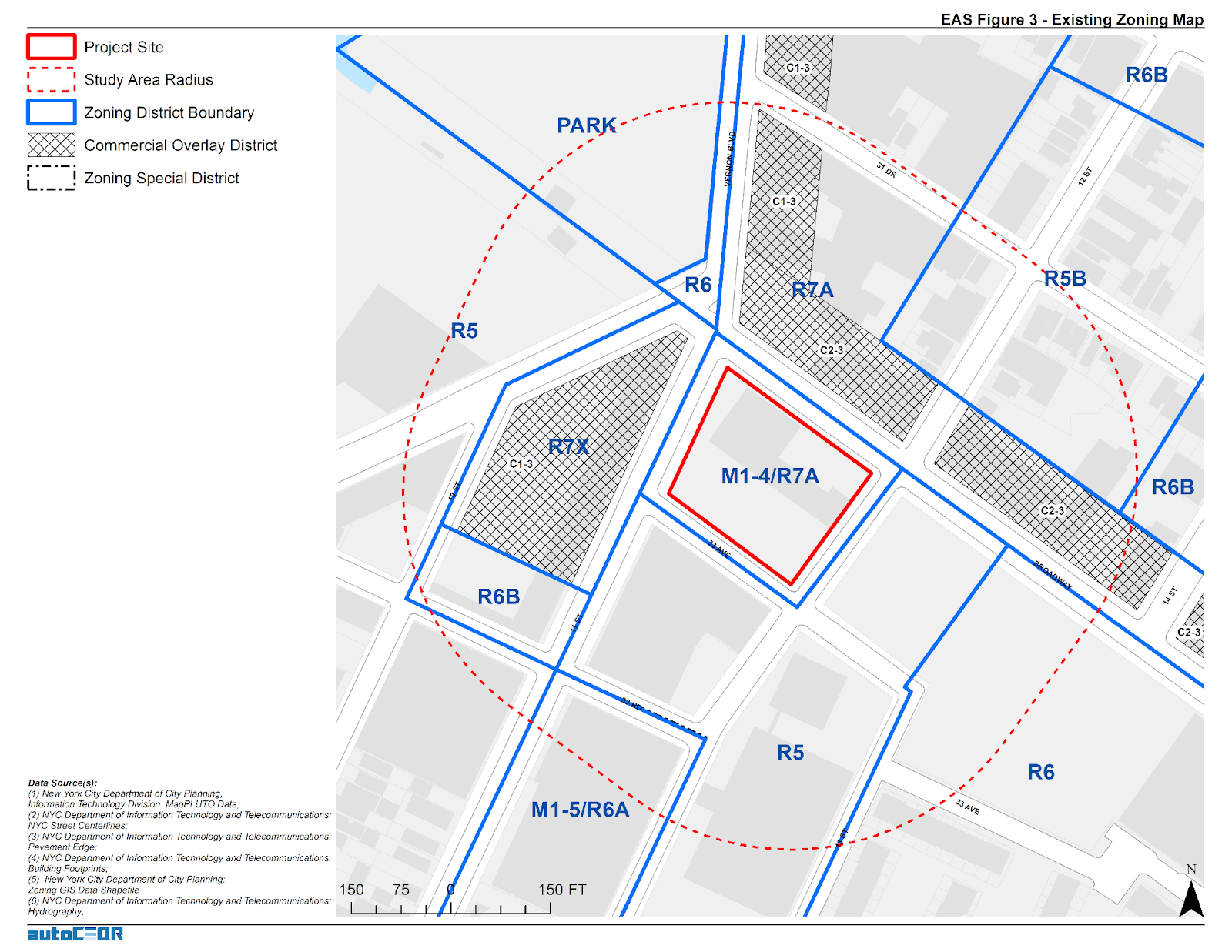
In addition to being consistent with existing property zoning classifications, projects are also reviewed in context of proximity to a myriad of other special districts and overlay zones.
B. Application Process
Users input relevant project-specific information (e.g., dwelling units, building height, square footage, etc.) via the AutoCEQR website. From there the application software ingests the data and checks it against public data sources – usually with some intermediate geoprocessing steps required – and then references the analysis thresholds stated in the Environmental Assessment Form (EAS) to determine which analysis the proposed project is required to undertake as part of the CEQR environmental review. For certain quantitative calculations, AutoCEQR has translated all of that logic into functions or classes in the codebase. Users also receive the data and maps for either a CEQR Pre-Screen or a select set of CEQR Full Analysis items. This VIMEO video provides an introduction to accessing the application and illustrates the products generated.
C. Usage
To date, AutoCEQR has had several dozen environmental professionals targeted from a few key firms to evaluate application and then go on to use AutoCEQR in production. Currently Sheeran and Sloane are allowing users to leverage AutoCEQR freely in order to get helpful product feedback and gain traction. With the aim of soliciting feedback for refinement, feature expansion, and product evolution, AutoCEQR has been well received by former director of the NYCDCP Environmental Assessment Review Division, Ms. Olga Abinader. She comments:
“AutoCEQR is an excellent application – as its title indicates, it automates tedious, time-consuming CEQR documentation that has historically taken consultants dozens of person-hours to complete. As a longtime NYC environmental review expert and former public service leader, I appreciate that it gathers data points from the City’s publicly available databases and agency websites (MapPLUTO, NYC Parks, NYC LPC, GIS sources), and combines this information with user inputs (i.e., analysis framework details) to generate useful EAS Maps, visuals, and content/data for the EAS Forms in a short turnaround. Given the time savings it offers, I am very enthusiastic about AutoCEQR as a tool and recommend it highly to consultants, public service professionals, the general public, decision-makers and others interested in preparing or reviewing CEQR materials.”
As the product is currently operating under a freemium model, users don’t need to currently apply the discount. However, it is important for AutoCEQR to continue this offering to support affordable housing in NYC in the event AutoCEQR ever moves to any kind of fee-based model.
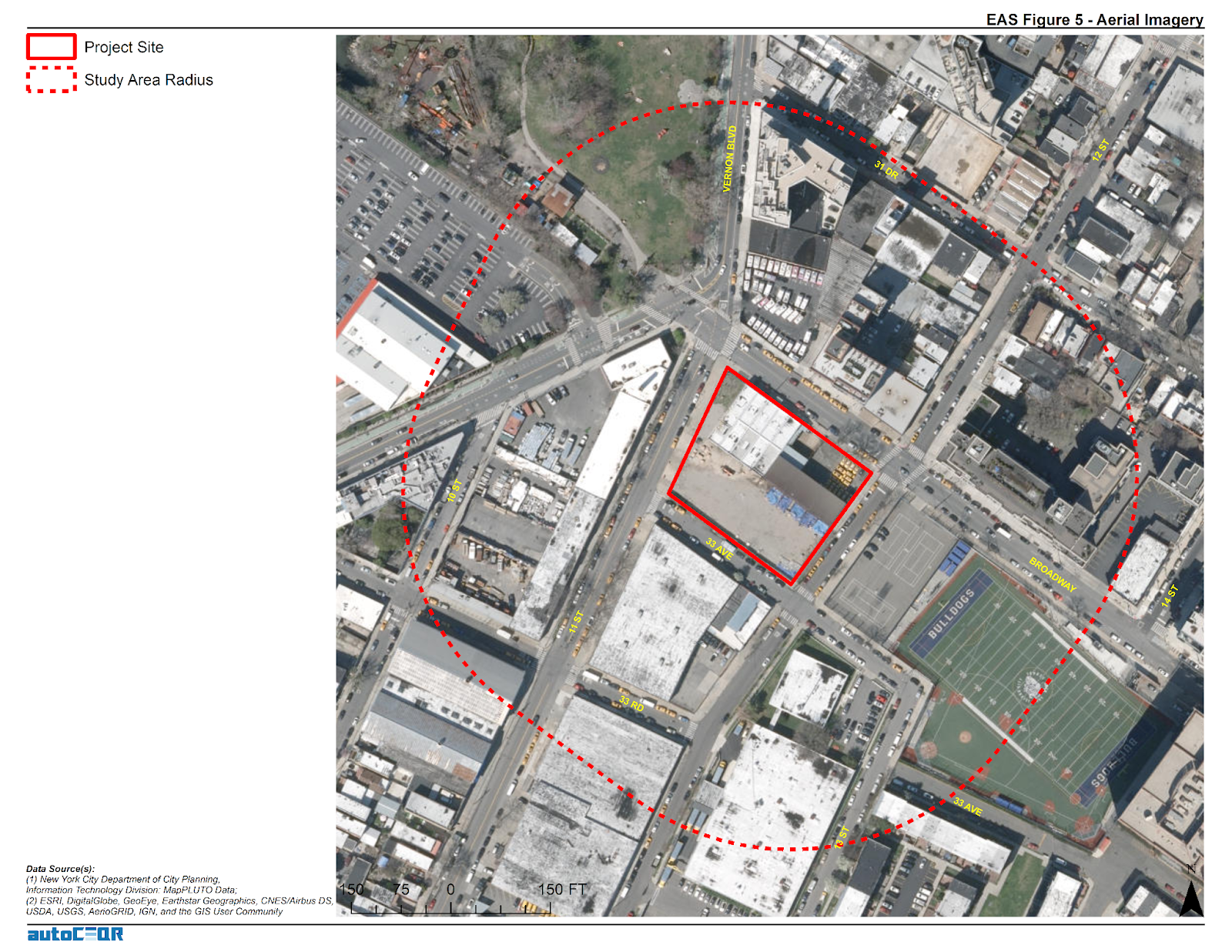
All AutoCEQR maps included in the project delivery file as both ArcGIS Map Document files (.mxd) and Map Package files (.mpk).
D. Affordable Housing Development Services Discount
Those working on the development of Affordable Housing or Inclusionary Housing are encouraged to contact the AutoCEQR team. It is their aim is to provide the AutoCEQR platform and reporting deeply discounted for individuals or companies involved in these types of housing projects. If the entire development provides 100% Affordable units, the AutoCEQR team intends to provide free reporting and analysis.*
As the product is currently operating under a freemium model, users don’t need to currently apply the discount. However, it is important for AutoCEQR to continue this offering to support affordable housing in NYC in the event AutoCEQR ever moves to any kind of fee-based model.
* Free reporting with minimal overhead for costs associated with report processing.
Summary
Development and marketing efforts on the AutoCEQR project has slowed down since both Sheehan and Sloane have started new full-time positions. Nonetheless, both continue to explore interesting options for its future development and continued success. Individuals and companies interested in the application and/or communicating with Sheehan and Sloane are encouraged to do so via the contact information below.
Contact:
Daniel M. Sheehan
danny@autoceqr.com
Matt Sloane
matt@autoceqr.com